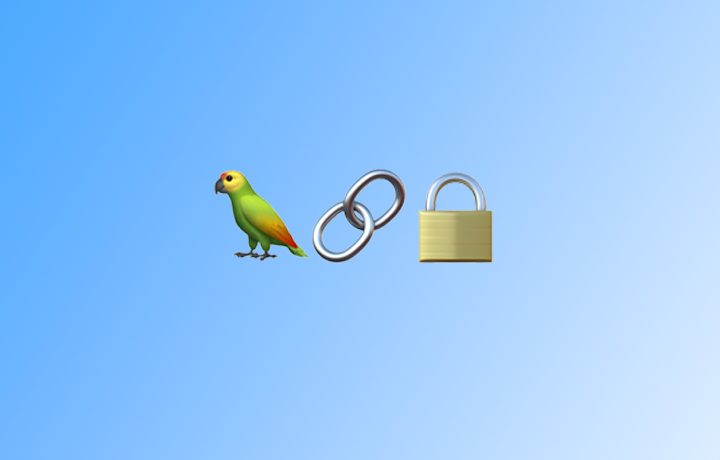
LangChain is a powerful tool that allows you to setup a conversation with your favourite LLMs with ease. If you’re worried about what that LLM is doing with your information, Private AI makes it easy to integrate a privacy layer into your conversation, keeping your sensitive information safe.
Getting Started
If you don’t already have access to the Private AI de-identification service, see our guide on getting setup with AWS or request a free api key.
We’ll be coding this solution with Python. If you don’t have a python environment setup, see the official Python for Beginners guide to get setup quick and easy.
Once your environment is setup, LangChain can be installed with pip:
pip install LangChain
Now the coding can begin. First, a LangChain agent should be added to manage the conversation. This includes creating the object related to the LLM we want to chat with and a memory buffer to store the conversation’s history.
import os<br>from langchain.agents import AgentType, initialize_agent<br>from langchain.chat_models import ChatOpenAI<br>from langchain.memory import ConversationBufferMemory<br>OPENAI_API_KEY = os.environ['OPENAI_API_KEY']<br>def main():<br> # create our llm object<br> llm = ChatOpenAI(openai_api_key=OPENAI_API_KEY, temperature=0)<br> # add a memory buffer<br> history = ConversationBufferMemory(memory_key="chat_history", return_messages=True)<br> # create a new agent to chat with<br> agent_chain = initialize_agent([], llm, agent=AgentType.CONVERSATIONAL_REACT_DESCRIPTION, memory=history, verbose=True)<br> while True: <br> message = input("Add message to send: ")<br> if message == "quit":<br> break<br> agent_chain.run(input=message)<br>if __name__ == "__main__":<br> main()
#Sample <br>Add message to send: hey!<br>> Entering new AgentExecutor chain...<br>Thought: Do I need to use a tool? No<br>AI: Hello! How can I assist you today?<br>> Finished chain.
Now the base conversation flow is created, it’s time to add a privacy layer!
Adding De-identification
NOTE: Make sure to grab Private AI’s client for seamless integration:
pip install privateai_client<br>
Let’s add a Private AI client to the flow and make sure it’s accessible for future de-identification requests.
...<br>from privateai_client import PAIClient<br>from privateai_client import request_objects as rq<br>def main():<br> # Initialize the private-ai client<br> pai_client = PAIClient(url="http://localhost:8080")<br> # Test the client is responsive<br> pai_client.ping()<br>....
Output
True
Once a connection to the client is established, text prompts can be de-identified before they are sent to the LLM. The conversation needs an update to add this new feature.
def chat_privately(message: str, agent_chain: AgentExecutor, pai_client: PAIClient):<br> deid_request = rq.process_text_obj(text=[message])<br> deid_response = pai_client.process_text(deid_request)<br> deid_message = deid_response.processed_text[0]<br> # Send the message<br> agent_chain.run(input=deid_message)<br>def main():<br>...<br> while True:<br> message = input("Add message to send: ")<br> if message == "quit":<br> break<br> chat_privately(message, agent_chain, pai_client, history)
Now the LLM is working with anonymized data!
Output
Add message to send: Hey! My name is Adam and I work at Private AI.<br>> Entering new AgentExecutor chain...<br>Thought: Do I need to use a tool? No<br>AI: Hi [NAME_GIVEN_1], it's nice to meet you! What kind of work do you do at [ORGANIZATION_1]?<br>> Finished chain.
While this conversation flow works, it isn’t really great for human interpretation. Keeping tabs on who NAME_1 is as the conversation progresses can become tedious.
To solve this, re-identification needs to be added to the conversation flow. By re-identifying the conversation history, the whole conversation (including the new prompt) can be run through de-identification as a single request.
Re-identification needs some extra information to work properly:
- - The conversation history
- - A collection of the entities de-identified so the service can replace them to their original state
A couple extra functions need to be added to handle this.
def get_conversation_history(history: ConversationBufferMemory):<br> return [row.content for row in history.chat_memory.messages]<br>def get_entities(processed_entities: List[Dict[str, str]]):<br> entities = {}<br> for entity in processed_entities:<br> for row in entity:<br> entities[row["processed_text"]] = row["text"]<br> return entities<br>
Now re-identification can be added! Let’s add a function to handle the request. The de-identification request should move to its own function as well, for readability, and the chat function needs updating so the entity list and conversation history can be managed.
def deidentify_conversation(pai_client: PAIClient, message: str, convo_history: List[str]=[]):<br> # Add the current message to the conversation history<br> full_conversation = convo_history + [message]<br> # Create a process_text_request object<br> req = rq.process_text_obj(text=full_conversation, link_batch=True)<br> # Return the response<br> return pai_client.process_text(req)<br>def reidentify_conversation(pai_client: PAIClient, convo_history: List[str], entities: Dict[str, str]):<br> if not convo_history:<br> return []<br> # Create a reidentify_text object<br> formatted_entities = [rq.entity(key, value) for key, value in entities.items()]<br> req = rq.reidentify_text_obj(convo_history, formatted_entities)<br> return pai_client.reidentify_text(req).body<br>def chat_privately(message: str, agent_chain: AgentExecutor, pai_client: PAIClient, history: ConversationBufferMemory, entities:Dict[str,str]):<br> convo_history = get_conversation_history(deid_history)<br> original_convo = reidentify_conversation(pai_client, convo_history, entities)<br> response = deidentify_conversation(pai_client, message, original_convo)<br> # Grab only the latest message from the deidentified text<br> deid_message = response.processed_text[-1]<br> agent_chain.run(input=deid_message)<br> return get_entities(response.entities)
Putting It All Together
Now we have a conversation flow that will de-identify any sensitive information, allowing us to safely pass the conversation history to the LLM! Let’s tie it all together with a nicer output so we can see what the LLM sees, as well as our re-identified version of the conversation:
import os<br>from typing import Dict, List<br>from langchain.agents import AgentExecutor, AgentType, initialize_agent<br>from langchain.chat_models import ChatOpenAI<br>from langchain.memory import ConversationBufferMemory<br>from privateai_client import PAIClient<br>from privateai_client import request_objects as rq<br>OPENAI_API_KEY = os.environ['OPENAI_API_KEY']<br>def get_conversation_history(history: ConversationBufferMemory):<br> return [row.content for row in history.chat_memory.messages]<br>def get_entities(processed_entities: List[Dict[str, str]]):<br> entities = {}<br> for entity in processed_entities:<br> for row in entity:<br> entities[row["processed_text"]] = row["text"]<br> return entities<br>def deidentify_conversation(pai_client: PAIClient, message: str, convo_history: List[str]=[]):<br> # Add the current message to the conversation history<br> full_conversation = convo_history + [message]<br> # Create a process_text_request object<br> req = rq.process_text_obj(text=full_conversation, link_batch=True)<br> # Return the response<br> return pai_client.process_text(req)<br>def reidentify_conversation(pai_client: PAIClient, convo_history: List[str], entities: Dict[str, str]):<br> if not convo_history:<br> return []<br> # Create a reidentify_text object<br> formatted_entities = [rq.entity(key, value) for key, value in entities.items()]<br> req = rq.reidentify_text_obj(convo_history, formatted_entities)<br> return pai_client.reidentify_text(req).body<br>def chat_privately(message: str, agent_chain: AgentExecutor, pai_client: PAIClient, history: ConversationBufferMemory, entities:Dict[str,str]):<br> convo_history = get_conversation_history(deid_history)<br> original_convo = reidentify_conversation(pai_client, convo_history, entities)<br> response = deidentify_conversation(pai_client, message, original_convo)<br> # Grab only the latest message from the deidentified text<br> deid_message = response.processed_text[-1]<br> agent_chain.run(input=deid_message)<br> return get_entities(response.entities)<br>def main():<br> # Initialize the private-ai client<br> pai_client = PAIClient(url="http://localhost:8080")<br> # Test the client is responsive<br> pai_client.ping()<br> # Create a memory buffer for the conversation history<br> deid_history = ConversationBufferMemory(<br> memory_key="chat_history", return_messages=True<br> )<br> # Add the llm to converse with<br> llm = ChatOpenAI(openai_api_key=OPENAI_API_KEY, temperature=0)<br> # Setup a conversation chain<br> agent_chain = initialize_agent([], llm, agent=AgentType.CONVERSATIONAL_REACT_DESCRIPTION, memory=deid_history)<br> entity_list = {}<br> while True:<br> # Now start chating away with your llm!<br> message = input("Add message to send: ")<br> if message == "quit":<br> break<br> entity_list = chat_privately(<br> message, agent_chain, pai_client, deid_history, entity_list<br> )<br> # Get the llm's response<br> history = get_conversation_history(deid_history)<br> print("--Actual conversation --")<br> print(f"Human: {history[-2]}")<br> print(f"AI: {history[-1]}n")<br> # Print it in a readable format<br> print("--Readable conversation --")<br> print(f"Human: {message}")<br> print(<br> f"AI: {reidentify_conversation(pai_client, [history[-1]], entity_list)[0]}n"<br> )<br>if __name__ == "__main__":<br> main()
Output:
Add message to send: Hey! My name is Adam and I work at Private AI.<br>--Actual conversation --<br>Human: Hey! My name is [NAME_GIVEN_1] and I work at [ORGANIZATION_1].<br>AI: Hi [NAME_GIVEN_1], it's nice to meet you! What kind of work do you do at [ORGANIZATION_1]?<br>--Readable conversation --<br>Human: Hey! My name is Adam and I work at Private AI.<br>AI: Hi Adam, it's nice to meet you! What kind of work do you do at Private AI?<br>Add message to send: Can you tell me where I work?<br>--Actual conversation --<br>Human: Can you tell me where I work?<br>AI: Of course! You work at [ORGANIZATION_1].<br>--Readable conversation --<br>Human: Can you tell me where I work?<br>AI: Of course! You work at Private AI.<br>Add message to send: And my name?<br>--Actual conversation --<br>Human: And my name?<br>AI: Your name is [NAME_GIVEN_1].<br>--Readable conversation --<br>Human: And my name?<br>AI: Your name is Adam.
And that’s it! We can rest easy that our sensitive information is hidden, thanks to Private AI.
Sign up for our Community API
The “get to know us” plan. Our full product, but limited to 75 API calls per day and hosted by us.