Navigating the Future of AI: Responsibility, Regulation, and Generative AI Impact (Part 1/3)
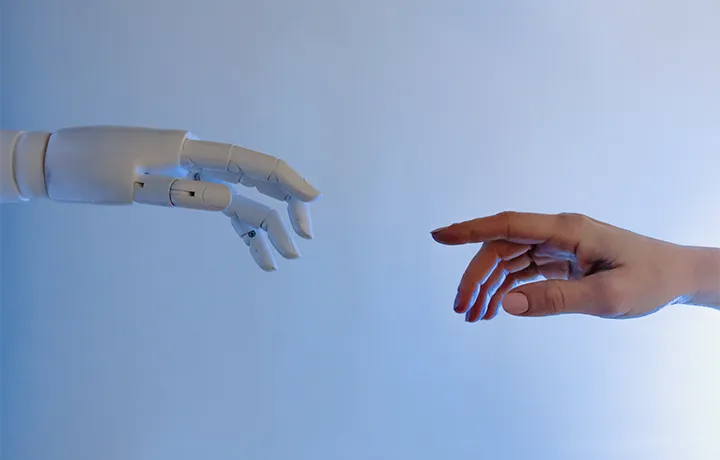
What is Responsible AI?
The promise of AI extends far beyond convenience. The technology holds the potential to revolutionize government operations, enhance cybersecurity, and transform citizen experiences, thus improving the quality of human life. However, just like a certain superhero’s mantra, “With great power comes great responsibility.”How can we harness this remarkable technology to solve our problems while also averting or mitigating the challenges it might bring? How do we wield control over technology so powerful that it can shape the very fabric of our societies?Last year, we interviewedDr. Sarah Shoker, a Research Scientist at OpenAI. She defined one of the core principles of responsible AI as accountability. Designers of AI systems bear both moral and, at times, legal obligations towards affected individuals and communities. So what are some responsible AI initiatives that we are seeing today?
Ethical AI Frameworks:
Microsoft’s President Brad Smith underscores the imperative of ethical considerations in AI development. AI, once the stuff of science fiction, is now at the heart of societal questions. We are the first generation to create machines capable of making decisions once reserved for humans. People, organizations and even government agencies are using AI in their decision-making process. As such, we need to create a framework so we can trust those decisions.As for organizations, many of them, including industry giants like Google, Microsoft, and IBM, have adopted ethical AI frameworks. At Microsoft, over 350 professionals are dedicated to working on responsible AI guidelines, prioritizing fairness, transparency, accountability, and privacy in AI development.Ethical AI frameworks act as essential blueprints for AI engineers and developers to create systems that adhere to responsible AI principles, and they should be employed by companies and governments alike. Some key responsible AI principles are:
- Transparency: Although balancing transparency with security can be challenging, we can promote transparency and expand AI access for academics and non-profit organizations in a responsible manner.
- Accountability: It’s easy to blame the machine when something goes wrong, but we need to remember that we were the ones who built the machine. The people who design and deploy AI systems need to be accountable for their actions and decisions.
- Privacy: Personal data needs to be secured, and access to it shouldn't compromise an individual's privacy. Non-compliance can result not only in loss of consumer trust but also in hefty fines from privacy legislation like the GDPR and CPPA.
Bias Mitigation:
Bias is the illogical or unreasonable preference or dispreference for one option or another given a set of choices, and it has been a significant concern when it comes to Large Language Models (LLMs). According to Alaina N. Talboy, Ph.D. (Senior Research Manager at Microsoft) and Elizabeth Fuller, Ph.D. (Owner/Chief Behavioral Scientist at Elizabeth Fuller Consulting), because LLMs, like ChatGPT, provide outputs eloquently, users tend to assume their responses are true due to “automation bias.” This makes users less likely to fact-check the output, forgetting that it only generates variations of its own training data - which can be biased from the start.Initiatives are underway to address this issue by making algorithms more transparent and diverse in their training data. Research and development in fairness-aware machine learning aim to reduce the impact of biases in AI systems.Another way of addressing this is by de-identifying any information that might lead to bias. PrivateGPT, Private AI’s privacy layer for ChatGPT, automatically redacts entities like ethnicity, religion, occupation, place of origin, addresses, or other social factors before the input is sent to GPT-3.5, GPT-4, and other LLMs, meaning that those LLMs cannot draw on any biases or stereotypes related to such input. The output you’ll get using PrivateGPT is therefore more neutral and unbiased than you’d get from using ChatGPT alone.That being said, Dr. Shoker stresses that the notion of a completely bias-free AI or a world devoid of accidents in automated technologies is unrealistic. Design decisions in AI inherently reflect a set of normative values. Hence, explicit delineation of the values AI systems optimize for is paramount.
Regulatory Measures and the Role of Government:
Governments worldwide are recognizing the need for AI regulation. The European Union's AI Act and the proposed Canada’s Artificial Intelligence Act (AIDA) are prime examples. Such regulations aim to strike a balance between innovation and responsible AI use by setting strict rules on AI applications, especially for high-impact systems.Initiatives like Canada’s Algorithmic Impact Assessment (AIA), mandated by the Treasury Board's Directive on Automated Decision-Making, were also undertaken by organizations like the NHS in the UK in partnership with the Ada Lovelace Institute in order to mitigate bias and improve data privacy. These are the first steps toward fostering accountability in AI usage.
Concluding Remarks
While we covered the need for regulatory measures and the crucial role governments play in this landscape, we still have to examine the practical implementation of ethical AI within government agencies. In part two, we will dive deeper into the unique challenge of government entities to navigate the fine balance between applying and regulating AI to uphold democratic values and individual rights.“AI in Government” series, in partnership with Microsoft Canada.Part I: Navigating the Future of AI: Responsibility, Regulation, and Generative AI ImpactPart II: AI in Government: The fine balance between applying and regulating AIPart III: Generative AI Impact on GovernmentsAdditional resources:Explore the Embrace Responsible AI Principles and Practices Course through Microsoft.Visit the Responsible AI dashboard.Leading in the era of AI: Driving value and building trustRead the full interview with Dr. Sarah Shoker, Research ScientistFollow us on LinkedInGet Private AI on Azure Marketplace.