Solutions – LLM
The Privacy Layer for LLMs
Unlock your data by rapidly removing and replacing personal and sensitive data from unstructured text, documents, audio, and images before training, fine-tuning, RAG, and prompting LLMs.
Unlocking Data Requires Data Minimization
Data minimization is a core requirement of data protection regulations worldwide, from the EU’s GDPR to the US’s proposed Federal American Privacy Rights Act (APRA).
Data minimization means keeping only the personal data you need and removing all other personal data. What counts as personal data within data protection regulations is a very long list, which is why we help you become GDPR Ready by supporting 50+ entity types across 53 languages.
Where should data minimization be integrated?
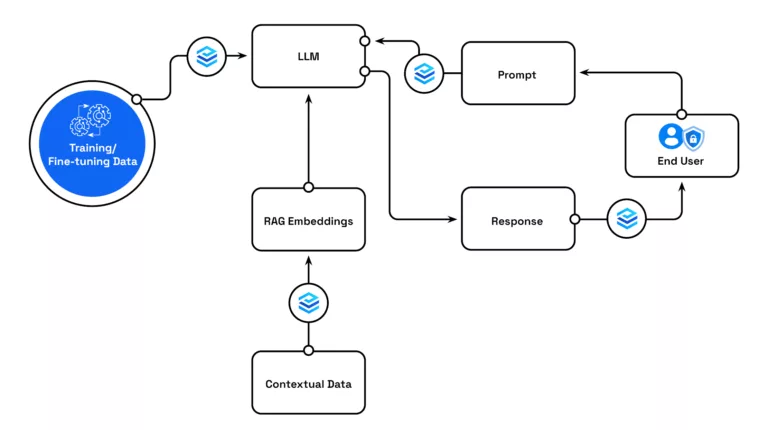
Data Minimization Best Practices

Sending Data to Third Parties
Teams want to innovate with the latest and greatest LLM technologies, but are blocked by their security teams. Private AI is trusted by security and privacy teams globally, giving them confidence to open up APIs to developers without losing control of their data.
Read: GDPR Compliance in the Life Cycle of LLM-Based Solutions
Customer Prompts & Inference
Prevent unwanted data from entering your prompt systems by redacting unnecessary and potentially risky information before the prompts are sent to the LLMs or logged anywhere (potentially creating compliance issues).
Training & Fine-Tuning LLMs
Train and fine-tune LLMs without “memorization” of sensitive information through context-and-utility-preserving anonymization.
Read: Fine-Tuning LLMs with a Focus on Privacy
Embeddings & Using RAG
Create safe embeddings by removing PII and PHI from source data used to create embeddings and from embeddings requests.
Read: Unlocking the Power of Retrieval Augmented Generation with Added Privacy: A Comprehensive Guide
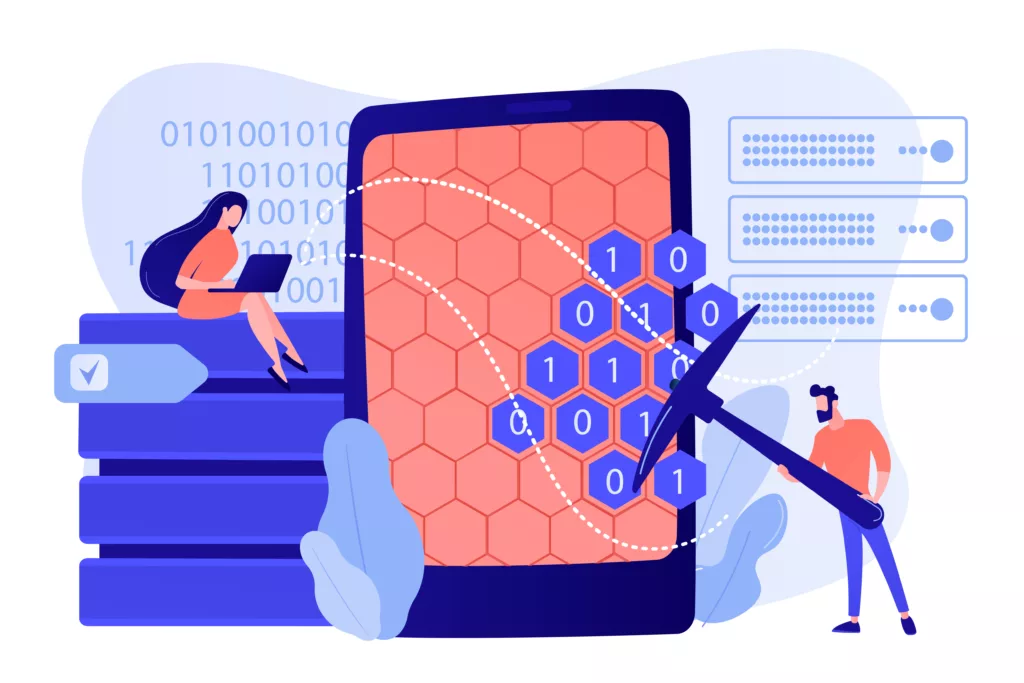
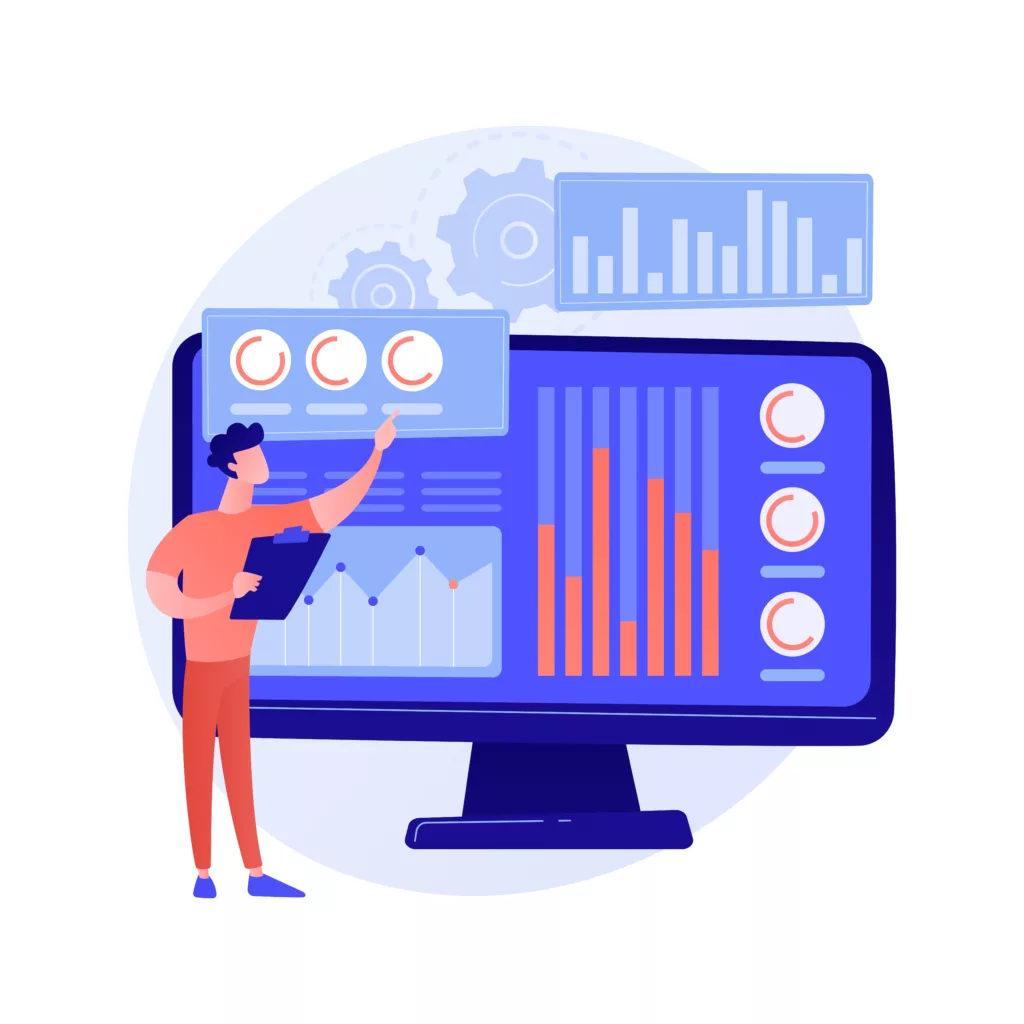
Output Sanitization
Continuous Monitoring
Data Privacy Built to Scale
Accuracy
We identify 50+ types of PII, PCI, and PHI across 52 languages, even if the language changes from one to another within a single document.
Enterprise Grade Security
Whether hosted in our Cloud, or deployed into yours, your data is never logged or used by us.
Speed
Private AI can process 70,000 words per second using our GPU-optimized models, or 2,000 words per second on a single core with our CPU-optimized models.
Customizable and Flexible
We offer full control over the platform. This includes which PII is identified, its deployment options, and the languages supported.
How the Privacy Layer for LLMs Works
For Developers
Integrate privacy into your LLM applications with just three lines of code. Replace sensitive data with entity labels, tokens, or synthetic information at training, fine-tuning, embeddings creation, and prompting stages. All of this with the added benefit of helping reduce bias in LLM responses by removing entities such as religion, physical location, and other indirect identifiers.
import openai
from privateai_client import PAIClient
from privateai_client import request_objects
@retry(wait=wait_random_exponential(min=1, max=60), stop=stop_after_attempt(6))
def chat_completion_with_backoff(**kwargs):
return openai_client.chat.completions.create(**kwargs)
def redact(raw_text):
request_obj = request_objects.process_text_obj(text=[raw_text])
response_obj = pai_client.process_text(request_obj)
return response_obj
def secure_completion(prompt, raw_text, temp):
######## REDACT DATA #####################
completions = {}
response_obj = redact(raw_text)
######## BUILD LOCAL ENTITIES MAP TO RE-IDENTIFY ########
deidentified_text = response_obj.processed_text
completions['redacted_text'] = deidentified_text
entity_list = response_obj.get_reidentify_entities()
######## SEND REDACTED PROMPT TO LLM #####
MODEL = "gpt-4"
completion = chat_completion_with_backoff(
model=MODEL,
temperature=temp,
messages=[
{"role": "user",
"content": f'{prompt}: {deidentified_text}'}
]
)
completions["redacted_completion"] = completion.choices[0].message.content
######## RE-IDENTIFY COMPLETION ##########
request_obj = request_objects.reidentify_text_obj(
processed_text=[completion.choices[0].message.content], entities=entity_list
)
response_obj = pai_client.reidentify_text(request_obj)
completions["reidentified_completion"] = response_obj.body[0]
return completions
For Security Teams
Set up Federated Control over each LLM workflow within your organization.
A control panel allows you to determine which products, teams, or employees can send which types of personal and sensitive data to LLMs, even based on your pre-existing access control settings.
Easy API integration allows you to send detection events to any monitoring system of your choice.

For End-users
PrivateGPT allows end users to interact with LLMs without worrying about their personal information being stored and used by third-parties.
